Let’s focus on the Octoparse Web Scraping tool, which helps us quickly fetch data from any website without coding techniques and anyone can use this tool to build a crawler in just minutes as long as the data is visible on the web page. If you asked me in short words about this tool, I would say this is a “No-code (or) Low-code web scraping tool.”, It takes really substantial time and be good to cope with a web-scraping. Since most companies are busy maintaining a business, data related services with low-code web scraping tools for a better choice to improve their productivity.
Ultimately the primary reason always is that it saves time across all industries. Certainly, everyone can take the advantage of the interactive workflow and intuitive tips guide to build their own scrapers.
Octoparse can fulfil most of the data extractions requirements to scrape the data from different websites like E-commerce, Social-Media, Structured and Tabulated pages. And it has capable of satisfying use cases like price monitoring, social trend discovery, risk management and many more.
There are many features that are there, let’s discuss a few major in this article.
Hardware and Software Requirements
To run Octoparse on your system and to use the easy web-scraping workflow, your system only needs to fulfil the following requirements:
Operating Systems:
- Win7/Win8/Win8.1/Win10(x64)
- Mac users can download the Mac version of Octoparse directly from the website.
Software
- Microsoft .NET Framework 3.5.
Internet Access
Environment of Octoparse
Let’s discuss the Octoparse environment, The Workspace is the place where we can build our set of tasks. There are four parts to it, each one plays its particular purpose.
- The Built-in Brower: Once you’ve entered a target URL page, the webpage will be loaded in Octoparse’s built-in browser. you can browse any website in the browse mode of operation, or you can click to extract the data you need in Select mode.
- The Workflow: To interact with the webpage(s), such as opening a web page, or clicking on a page element(s), the entire process is defined automatically in the form of a workflow.
- Tips Box: It uses smart Tips to “talk” to you during the extraction process and to guide you through the task building process.
- Data Preview: You can preview the data selected. It provided the option to rename the data fields or remove the undesirable items that are not needed.
The Octoparse installation package can be downloaded on the official website
How does Octoparse Work?
It automatically extracts the web page data by opening a web page and clicking the page like human browsing the page and starts extracting the data in a well-defined workflow and each action is pertaining to the target and objective of the purpose.
- Simulation
- Workflow
- Extraction
Understand the Octoparse Interface
Since Octoparse provides a very rich and user-friendly interface, anyone can do the data extraction from any web page. I could recommend the favoured task template would satisfy most of the tasks in a few minutes. It would be the data for analysis from various classifications like – Products, Travel, social media, Search Engines, Jobs, Real Estate and Finance.
The main tabs are New, Dashboard, Data Services, Tools, and Tutorials
Let’s explore each item quickly.
Home Page
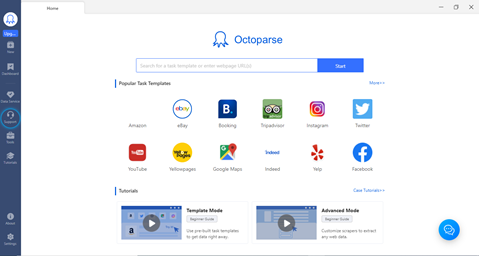
Workflow: The workflow-based design has been put in place in such a way, that it can be operated exclusively within GUI. Scripts or manual insertion of code is partly possible, but not necessary. In Octoparse Workflow there are two methods you can use that are nothing but Advanced Mode and the Template Mode.
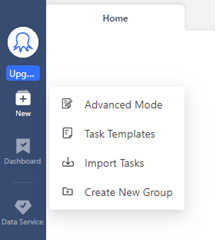
Task Templates: Which is used for pre-built tasks to get data by entering simple parameters like URL(s) or keywords. There are over 60+ templates for most mainstream websites. There is no need to build anything specifically and no technical chandelles. Simply select a template you need, check the sample data to see if it gets what you need, and extract the data right.
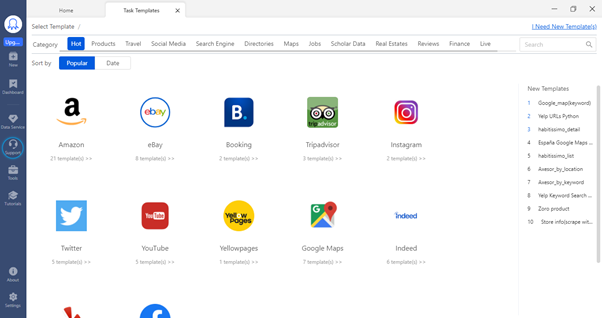
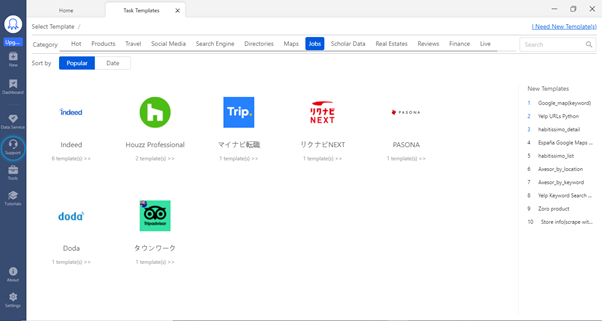
You can very well go into a group of templates specific to a country, based you can extract the data for use cases analysis.
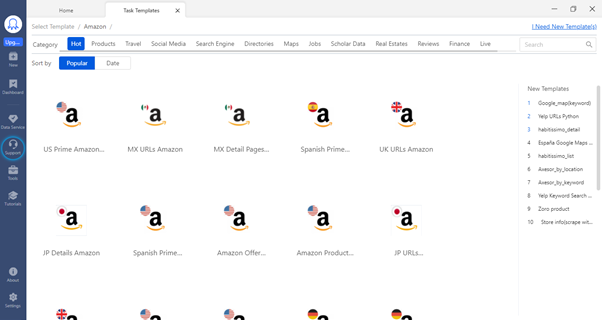
Templates Configuration
- Data preview: The data preview tab will help you to find out the list of items extracted during the process.
- Parameter: The parameter would help you to get the URL to run for your data extraction.
- Sample: This tab will give you the extracted data in tabular format.
- Advanced Mode: The Advanced Mode use to take overall control of every step of your web scraping project.
Description
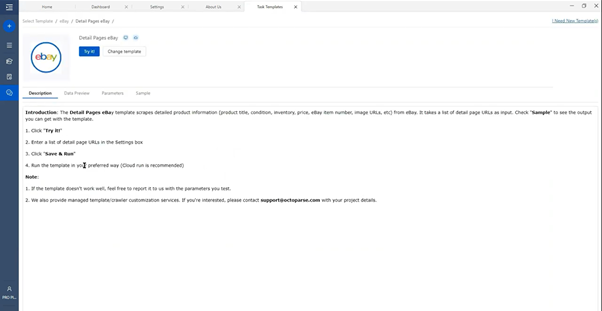
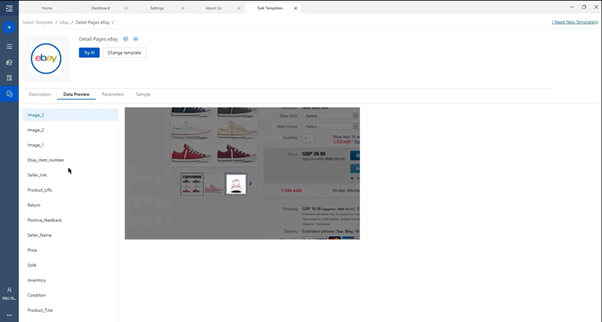
Extracted Data
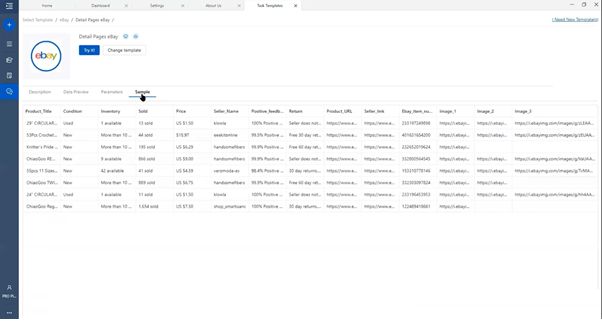
After a successful run, we could get all data in the tool and ready to further analysis.
Advanced Mode
Advanced Mode is a highly flexible and very powerful web-scraping mode than Task Templates. This is specifically for people who want to scrape from websites with complex structures for their project-specific.
The list of features of Advanced Mode:
- Achieve the scraping data on almost all kinds of webpage
- Extract data in different formats URL, image, and HTML
- Design a workflow to interact with a web page such as login authentication, keyword searching
- Customize your workflow, such as setting up a wait time, modifying XPath and reformatting the data extracted
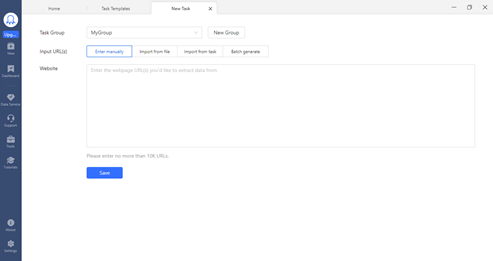
There is a provision for Edit or Create your Advanced Workflow, which you could explore with the tool explicit. Here you could simulate real human browsing actions, such as the below steps.
- Opening a web page
- Clicking on a page element or button to extract data automatically.
The whole extraction process is defined automatically in a workflow with each step representing a particular instruction in the scraping task.
Dashboard: You can manage all your scraping tasks. Rename, Edit, Delete and Organize all the tasks. You can also conveniently schedule any tasks.
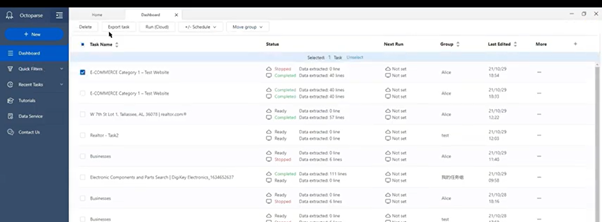
Extraction with the Octoparse Cloud
One of the nice features of Octoparse is a powerful Cloud platform for users can run their tasks 24/7. When you run a task with the “Cloud Extraction” option, it runs in the cloud with multiple servers using different IPs. Same time you can shut down your app or computer while the task is running. You need not worry about hardware and its limitation.
During this process data extracted will be saved in the cloud itself and can be accessed any time if you want. Here you can schedule the task, you can schedule your task to run as frequently as you need.
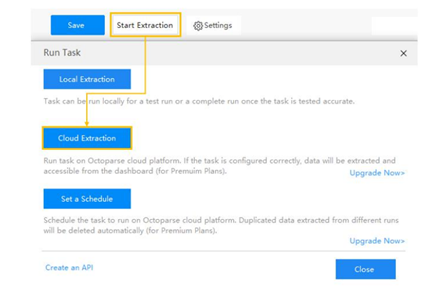
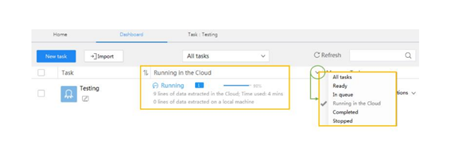
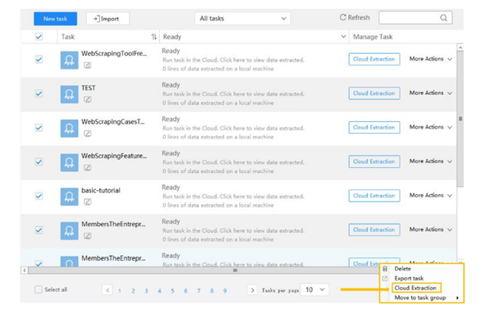
Auto-data export: The tool provided the Auto-Data export provision to export data to the database, and it can be automated and scheduled. There are multiple options to configure this feature and enhance more on the data export.
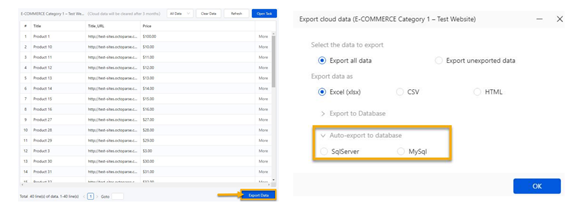
This tool also provides the refine of your data, like as below list of tasks:
- Rename/move/duplicate/delete a field
- Clean data
- Capture HTML code
- Extract page level-based data and date & time
You must know the Anti-Blocking settings which are available in this tool, few of them are below and you can very well add these to your workflow settings.
- IP Blocking:
- Since some websites might be very sensitive to web scraping and take some serious anti-scraping measures, by blocking IPs to stop any scraping activities.
- Browser recognition & Cookie Tracking:
- Every request made by a web browser is eventually blocked, due to the user-agent, With the help of this tool, you can easily enable automatic user-agent rotation in your crawler to reduce the risk of being blocked.
Data Services: where you and your team of web scraping experts can build the whole web scraping process customized for your needs.
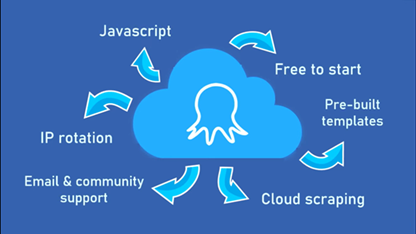
Conclusion
Guys, so far we have explored what is Web Scraping, Crawler in detail and the scope of both techniques and their significance during the data preparations stage, then we focused on the Octoparse tool and its key features right from its Hardware and Software Requirements, the Environment of Octoparse, How Octoparse works, Understanding of the Octoparse Interface, Key components – Workflow, Dashboard and Data Services, Extraction with the Octoparse are high demand, especially the Auto-data export – IP Blocking features are really major milestones during the process. Undoubtedly, this too would fulfil most of the data extractions requirements to scrape the data from different websites and always is that it saves time. Since the tool supports over 60+ predefined templates for most mainstream websites our job would be very simple. Hope you got the high-level details of the Octoparse tool and its benefits. You can very well install the same and explore more. Thanks for your time on this Web Scraping article.