In this problem statement, a classifier would be trained to find the gender (male or female) by providing the names. We need to use a heuristic to construct a feature vector and train the classifier. We will be using the labeled data from the scikit-learn package.
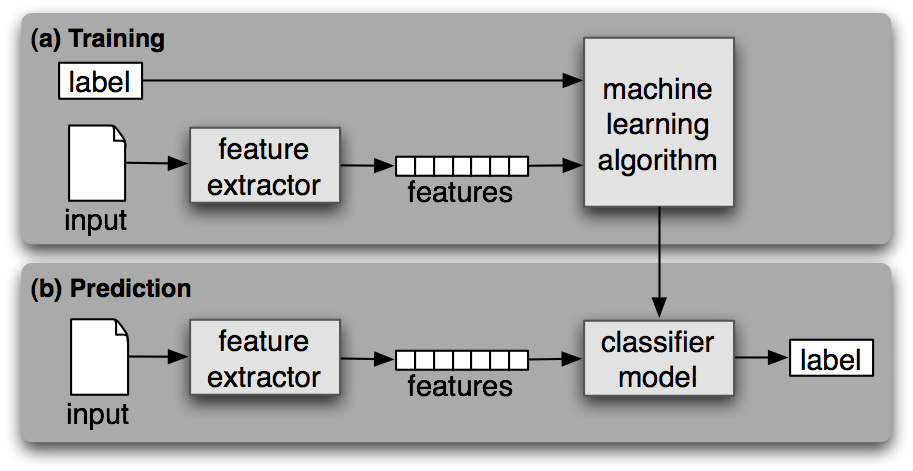
Following is the Python code to build a gender finder:
Let us import the necessary packages:
import random
from nltk import NaiveBayesClassifierfrom nltk.classify import accuracy as nltk_accuracy
from nltk.corpus import names
Now we need to extract the last N letters from the input word. These letters will act as features:
def extract_features(word, N=2):
last_n_letters = word[-N:]return {'feature': last_n_letters.lower()}
if __name__=='__main__':
Create the training data using labeled names (male as well as female) available in NLTK:
male_list = [(name, 'male') for name in names.words('male.txt')]
female_list = [(name, 'female') for name in names.words('female.txt')]data = (male_list + female_list)
random.seed(5)
random.shuffle(data)
Now, test data will be created as follows:
namesInput = ['Rajesh', 'Gaurav', 'Swati', 'Shubha']
Define the number of samples used for train and test with the following code
train_sample = int(0.8 * len(data))
Now, we need to iterate through different lengths so that the accuracy can be compared:
for i in range(1, 6):
print('\nNumber of end letters:', i)
features = [(extract_features(n, i), gender) for (n, gender) in data]
print('\nNumber of end letters:', i)
features = [(extract_features(n, i), gender) for (n, gender) in data]
train_data, test_data = features[:train_sample], features[train_sample:]
classifier = NaiveBayesClassifier.train(train_data)
classifier = NaiveBayesClassifier.train(train_data)
The accuracy of the classifier can be computed as follows:
accuracy_classifier = round(100 * nltk_accuracy(classifier, test_data), 2)
print('Accuracy = ' + str(accuracy_classifier) + '%')
print('Accuracy = ' + str(accuracy_classifier) + '%')
Now, we can predict the output:
for name in namesInput:
print(name, '==>', classifier.classify(extract_features(name, i)))
print(name, '==>', classifier.classify(extract_features(name, i)))
The above program will generate the following output:
Number of end letters: 1
Accuracy = 74.7%
Rajesh -> female
Gaurav -> male
Swati -> female
Shubha -> female
Accuracy = 74.7%
Rajesh -> female
Gaurav -> male
Swati -> female
Shubha -> female
Number of end letters: 2
Accuracy = 78.79%
Rajesh -> male
Gaurav -> male
Swati -> female
Shubha -> female
Accuracy = 78.79%
Rajesh -> male
Gaurav -> male
Swati -> female
Shubha -> female
Number of end letters: 3
Accuracy = 77.22%
Rajesh -> male
Gaurav -> female
Swati -> female
Shubha -> female
Accuracy = 77.22%
Rajesh -> male
Gaurav -> female
Swati -> female
Shubha -> female
Number of end letters: 4
Accuracy = 69.98%
Rajesh -> female
Accuracy = 69.98%
Rajesh -> female
Gaurav -> female
Swati -> female
Shubha -> female
Swati -> female
Shubha -> female
Number of end letters: 5
Accuracy = 64.63%
Rajesh -> female
Gaurav -> female
Swati -> female
Shubha -> female
Accuracy = 64.63%
Rajesh -> female
Gaurav -> female
Swati -> female
Shubha -> female
In the above output, we can see that accuracy in maximum number of end letters are two and it is decreasing as the number of end letters are increasing.
0 comments:
Post a Comment